CAI unites talent and technology to shape the future
What is your biggest challenge?
CAI powers the possible for business and industry progress
View all servicesTabbed content
Translating state and local government demands into transformational technology solutions
Between artificial intelligence guidelines, cybersecurity insurance and increased cyber threats, and demands to modernize with limited resources, CAI has helped clients navigate the constantly evolving technology landscape for over 40 years.
CAI offers innovative solutions for commercial organizations
We specialize in tailored business solutions that deliver real results. Unique marketplace demands deserve distinctive/exceptional solutions – solve for tomorrow with our end-to-end services today.
Reinventing how people, processes, and technology accelerate your business
With the right approach and planning, CAI’s Contingent Workforce Solutions can help you improve speed-to-hire and operational performance, lower labor costs, align staffing with business objectives, and increase transparency across contingent workforce management and expenditure.
Stay informed with CAI’s latest resources
View all resourcesCAI partners with NACo to advance AI in the public sector
To explore the applications, benefits, and risks of AI, CAI partnered with the National Association of Counties (NACo) to advance AI adoption in the public sector.
Read the success story →The benefits of business analysis, and why organizations should outsource it
Improving operational agility can be a straightforward endeavor with a dedicated service provider.
Read the thought leadership →Driving safety forward with the modernization of crash data reporting
A southern DOT turned to CAI for the creation of a custom system to streamline crash data reporting.
Read the success story →CAI revolutionizes industries with our expertise
View all industries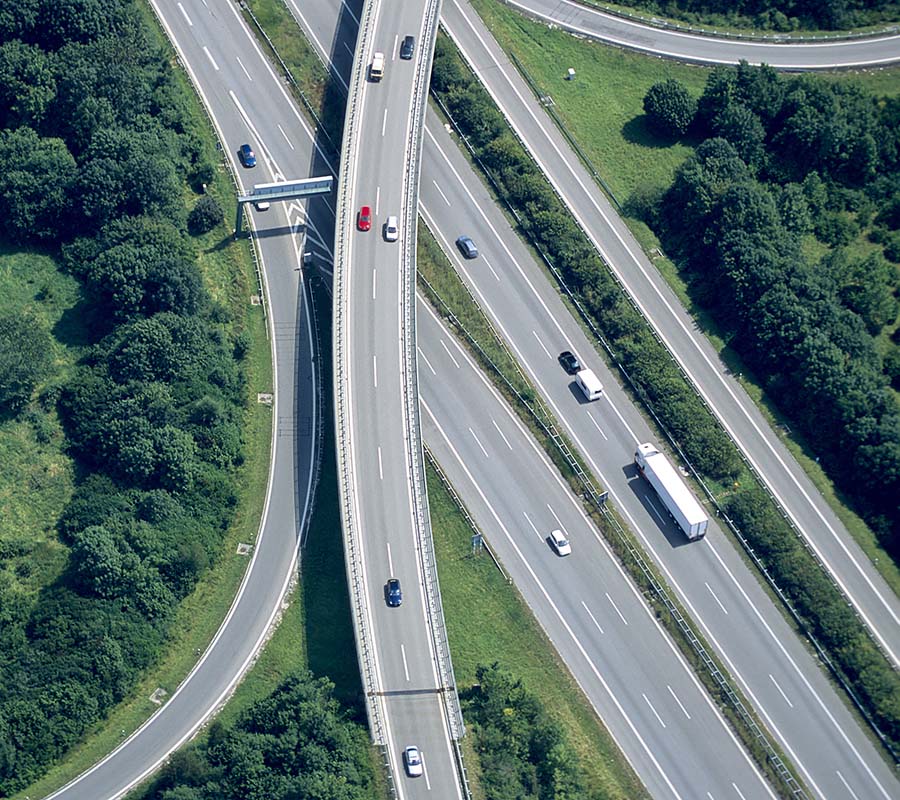
Transportation and Tolling
Transforming operations and technology with visibility and vision
Learn more →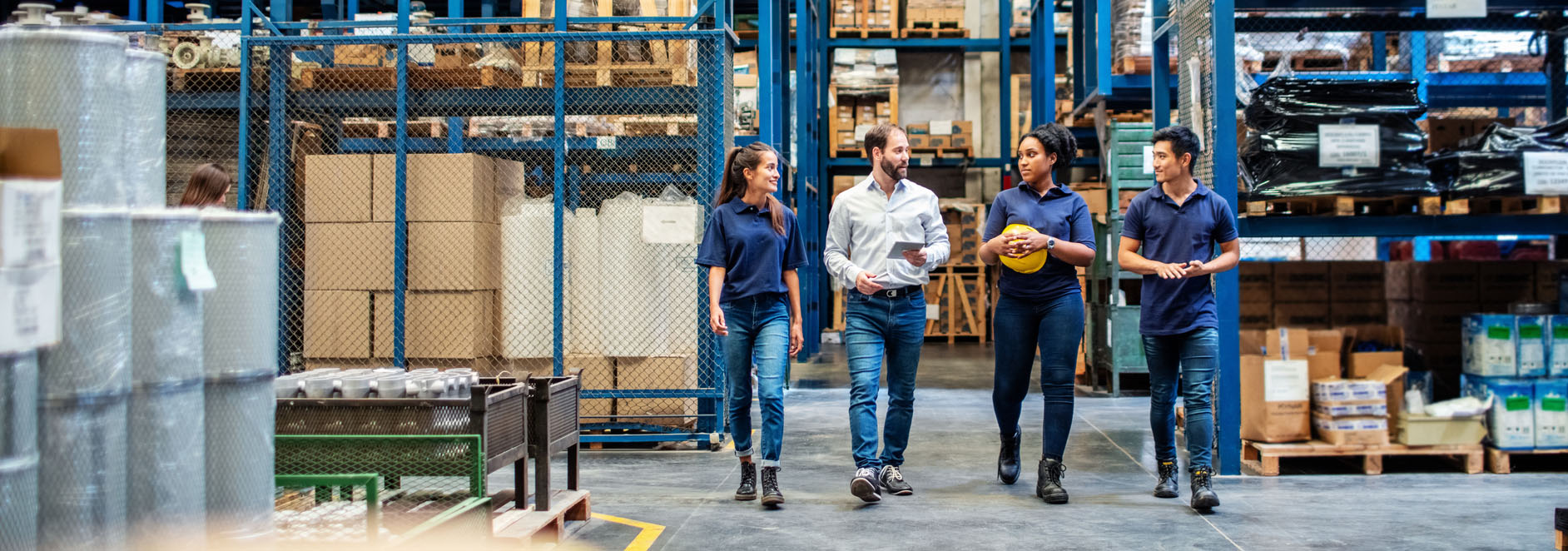
Manufacturing and Supply Chain
Increase transparency and connect with customers early, often, and always
Learn more →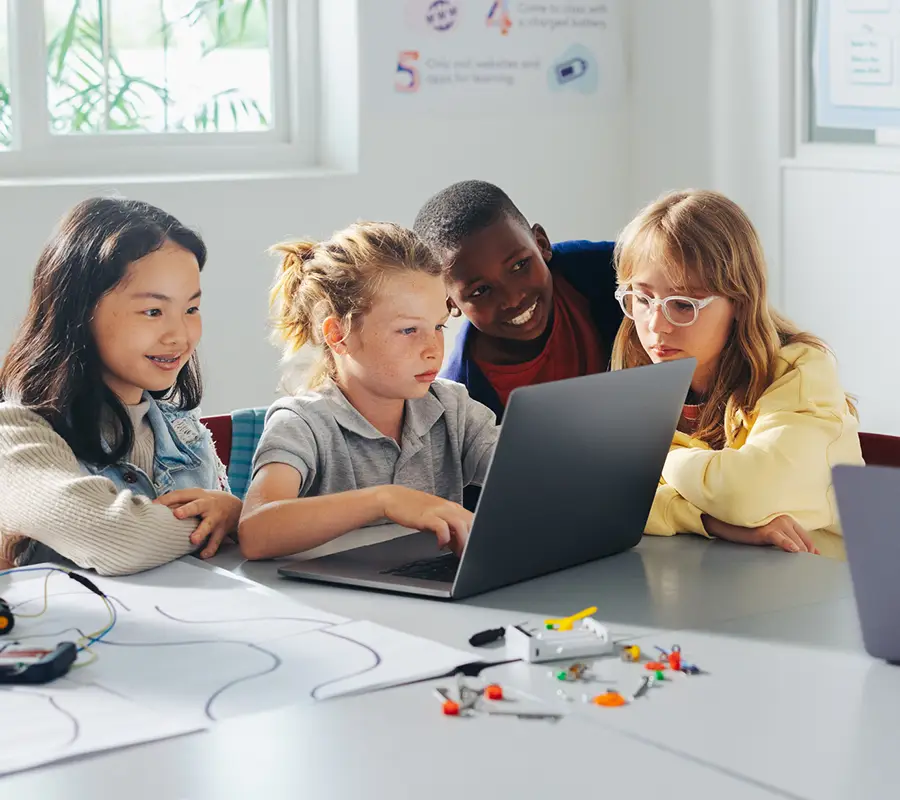
Education
Enhancing learning through engaging, inclusive, and secure educational environments
Learn more →Express links
Explore our resource library
Expand your interests with articles, thought leadership, webinars and more.